Background
The ‘lemon sign' refers to the inward scalloping of the frontal bones in a fetal skull and has a strong clinical association with multiple anomalies such as open neural tube defects, encephalocele, etc. The automated detection of lemon sign from ultrasonography (USG) scans can assist novice sonographers and clinicians in low-resource settings in providing timely and informed referrals to tertiary/specialist centers for further examinations. In this study, we design and validate a fully automated artificial intelligence (AI) system to detect lemon sign from 2D USG images of the fetal brain.
Methods
A total of 5791 USG images (normal/lemon sign cranium:4710/1081) of the transventricular (TV) and transcerebellar (TC) planes were retrospectively obtained from 1192 pregnancies (lemon sign: 44 pregnancies) through targeted mid-trimester USG examination at 2 tertiary referral centers using 3 commercially available USG devices (General Electric [GE] Healthcare; GE Voluson E8/P8/S10). We developed two AI networks to (1) identify the fetal cranium and obtain segmentation masks; (2) classify the segmentation masks as a lemon sign or normal. A U-Net based cranium segmentation network was trained and tested on 2400 and 719 images respectively. 'Enriched cranium segmentation masks’ (segmentation masks multiplied with latent space feature maps) were extracted for the remaining 2672 USG images using the trained segmentation network. A classifier network was trained and tested (equal number of lemon sign and normal cases) on the 800 and 1872 enriched cranium segmentation masks, respectively. The Dice coefficient was used to evaluate the performance of the cranium segmentation network (scale = 0: no-overlap; 1.0: complete overlap; comparison against manual segmentations). The sensitivity, specificity, and area under the receiver operating characteristics curve (AUC) were used for evaluating the performance of the classifier network. We also used GradCam maps to qualitatively analyze the important regions focused by the classifier to detect a lemon sign cranium and offer clinically interpretability of the AI network.
Results
The segmentation network achieved a Dice coefficient of 0.82. The normal/lemon sign classifier offered a sensitivity, specificity, and AUC of 0.88, 0.99, and 0.99. Qualitative analysis of the GradCam maps confirmed that the classifier network focused on the inward scalloping of the frontal bones in most cases.
Conclusion
The proposed AI system offers clinically interpretability and good performance in the fully automated detection of lemon sign fetal craniums. Its clinical translation to low-resource/remote settings can help sonographers provide timely referrals to specialists for detailed evaluation and management.
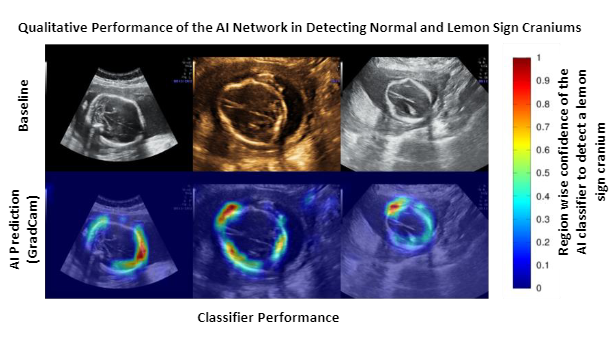
The second row represents the GradCam maps of the classifier for the baseline images.
The color scale on the right, indicates the region-specific confidence given by the classifier
(Blue = low confidence; Red = highest confidence) to detect lemon sign craniums.