A Multi-centre, Multi-Device Validation of a Deep Learning System for the Automated Segmentation of Fetal Brain Structures from Two-Dimensional Ultrasound Images
Objective
To validate (multicentre, multi-device) the robustness of a single deep learning (DL) system for the simultaneous and automated segmentation of 10 key fetal brain structures from multiple planes (transventricular [TV] and transcerebellar [TC]).
Methods
We retrospectively obtained 4,190 two-dimensional (2D) ultrasonography (USG) images (1349 pregnancies; TV + TC images) from 3 centres (2 tertiary referral centre [TRC 1,2] + 1 routine imaging centre [RIC]) using 6 ultrasound (USG) devices (GE Voluson: P8,P6,E8,E10,S10; Samsung: HERA W10). A custom U-Net was trained (2744 images from TRC 1 [E8, S10]) on 2D fetal brain images (TV + TC images) and their corresponding manual segmentations to segment 10 key fetal structures (TV + TC planes). We assessed the robustness (operator & centre variability) and generalisability (across devices) of the proposed approach across 4 independent (unseen) test sets. Test set 1 (TRC 1, trained devices): 718 images (E8, S10); test 2 (TRC 1, unseen devices): 192 images (HERA W10, P6, E10); test set 3 (TRC 2, trained device): 378 images (E8), and test set 4 (RIC, unseen device): 158 images (P8). The segmentation performance was qualitatively and quantitatively (Dice coefficient [DC]) assessed.
Results
Irrespective of the USG device/centre, the DL segmentations were qualitatively comparable to their manual segmentations. The mean (10 structures; test sets 1/2/3/4) DC were: 0.83 ± 0.09/0.80 ± 0.08/0.75 ± 0.09/0.80 ± 0.07.
Conclusion
The proposed DL system offered a promising and generalisable performance (multi centres, multi-device). Its clinical translation can assist a wide range of users across settings to deliver standardized and quality prenatal examinations.
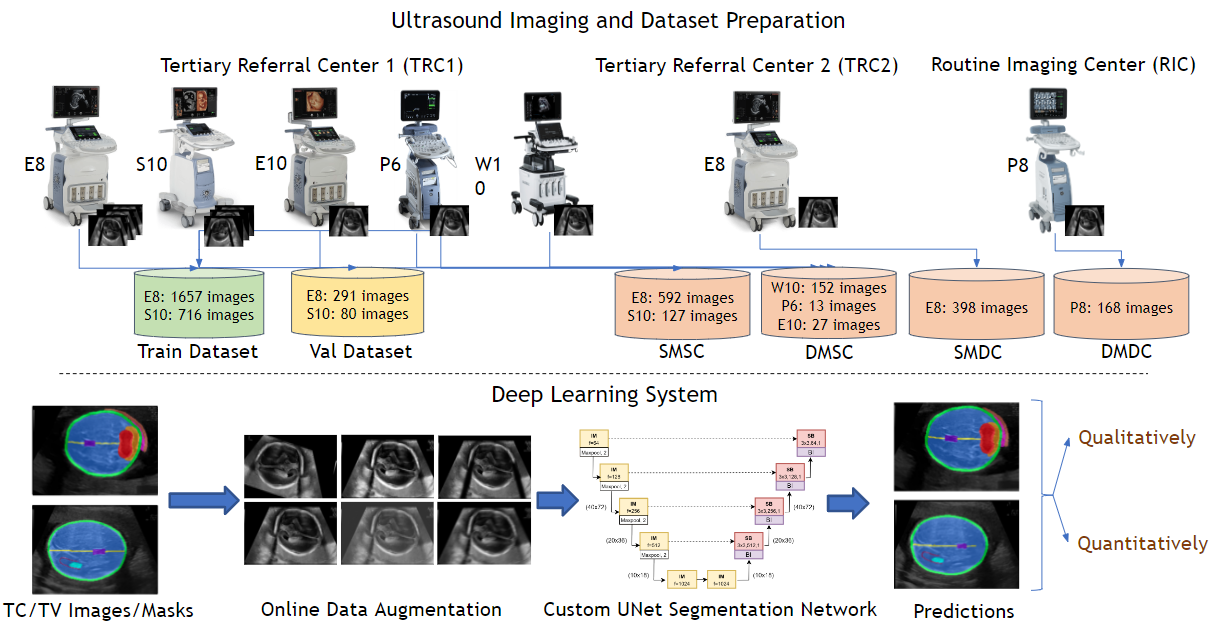
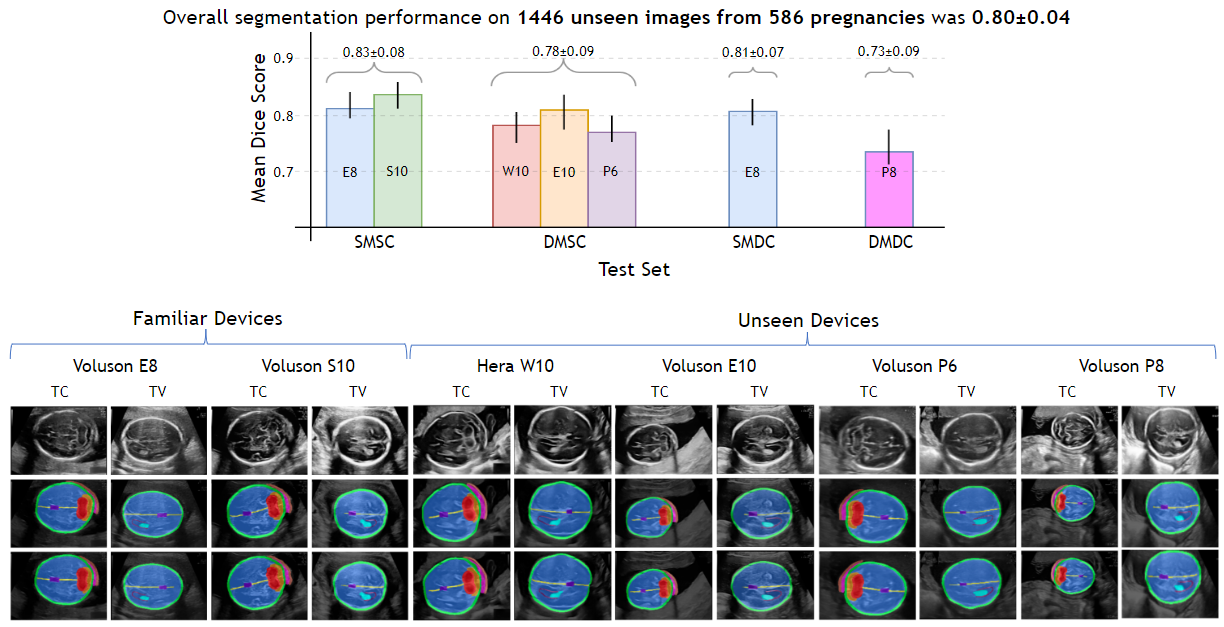